Data volumes are growing at an unprecedented rate. A new buzzword has thus entered the already crowded vocabulary of the data industry: the data lakehouse.
At ALTEN, we have already successfully set up data platforms with data lakehouses for our customers and will certainly continue to do so in the future. But what exactly is it all about?
Even the less data-savvy professionals have heard of data warehouses and data lakes, but now there is a new way to centrally store data for analytics purposes: The Data Lakehouse. To understand the concept of the data lakehouse, however, we first need to take a brief look at the history of analytic storage.
The origins of analytical storage
Since the beginning of the digital revolution in the second half of the 20th century, exponentially growing amounts of data have been processed. In the 1960s and 1970s, computers began to be used to handle transactional tasks in businesses, automating the recording that had previously been done manually.
However, as computing and storage technologies advanced, so did the amount of data, creating a problem for companies as they tried to analyze the data they were collecting. This is where the data warehouse comes in: a single-source-of-truth database in which a company’s data is centralized in separate databases optimized for analytical use. However, this structured storage of data also leads to a certain inflexibility. Schemas must be defined in advance, and implementing changes after the fact can be cumbersome, especially with large data sets.
From data lakes to data swamps
In the late 2000s and early 2010s, the volume of analytic data that organizations wanted to process exceeded the capacity of many traditional, on-premise data warehouses. The age of Big Data had arrived. To address these new challenges, the Data Lakes model was born.
Data Lakes can store data in any format and also support real-time data ingestion capabilities. Because of its advantages, the Data Lake quickly became the first choice for storing analytic Big Data. Unfortunately, however, efficiently implementing and managing a data lake is a Herculean task, and many data lakes have become data swamps due to a lack of management. These make data discovery almost impossible and hinder the successful extraction of analytical insights.
Combining the best of both worlds
In recent years, therefore, attempts have been made to reconcile the concept of the data warehouse and the data lake through the use of modern technologies. Ideally, the efficiency of the data warehouse and the flexibility of the data lake are combined in a scalable solution. It is from this ideal that the concept of the data lakehouse was developed and this is now being realized thanks to recent technological advances. Its strength can be seen in a number of key features, such as the ingestion and analysis of data in real time or the cost efficiency compared to modern data warehouses.
Of course, every new technology also brings its drawbacks. One of the main arguments currently being made against data lakehouses is that they are not yet as mature as their counterparts. Nonetheless, more and more companies have now adopted the lakehouse concept and found that it has matured to the point where it can deliver on its promise in production scenarios.
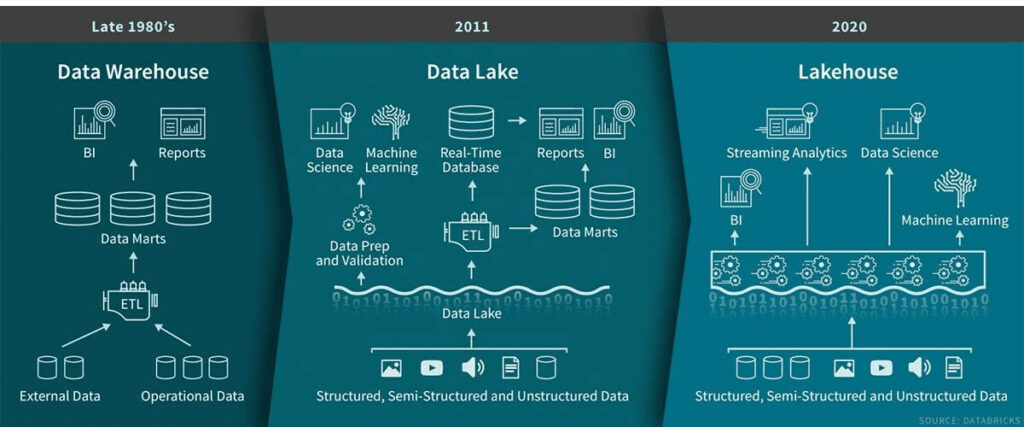
Conclusion
The data warehouse concept is very promising and represents a paradigm shift in terms of data platform architecture. Combining the high performance of data warehouses with the scalability and flexibility of data lakes provides the opportunity to leverage the best of both worlds. Of course, the data lakehouse concept has had some teething problems in the past, but fortunately it has evolved at an amazing pace that is expected to continue in the years to come. We at ALTEN are also sure that we will continue to set up such modern data architectures for our customers.